Last mile delivery within retail and ecommerce is complex and vital to get right. It impacts customer satisfaction and experience, repeat business, brand perception and profitability, among other things. Understandably, therefore, logistics and delivery planning teams tend to spend a significant amount of time preparing their delivery routes, developing the most cost-effective, environmentally friendly plans for their delivery teams and fleets to execute. Creating an optimised route plan for deliveries is the first step to maximising fleet performance and providing a positive customer experience.
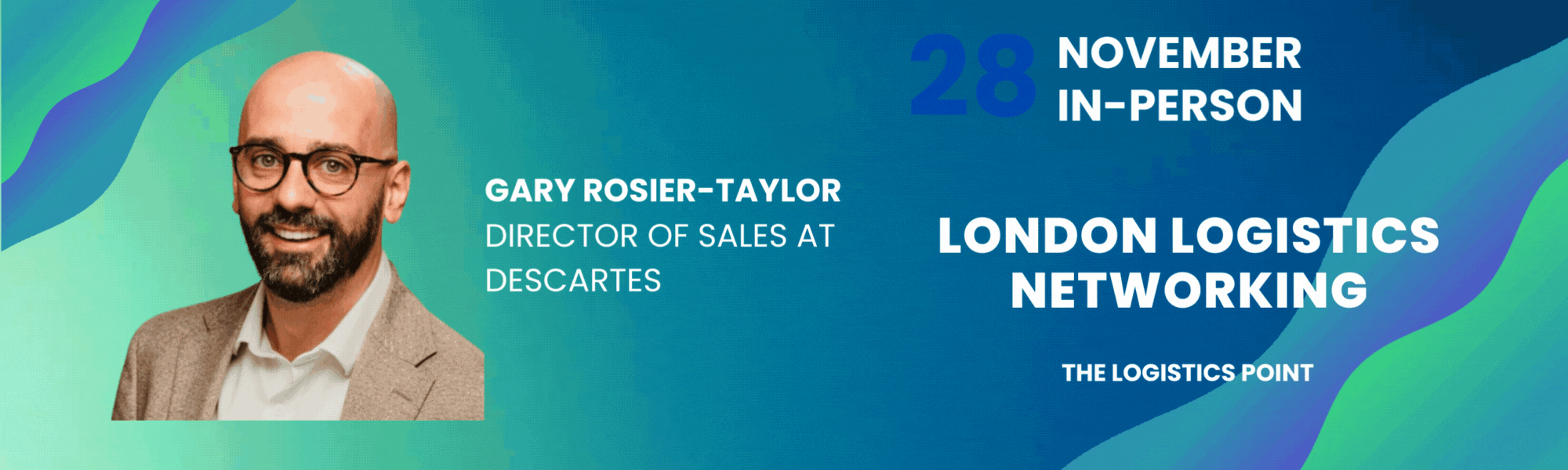
However, a great plan only matters when it is executed – and that’s the challenge for many fleet operators. Sure, tracking drivers with GPS helps, but the problem is not that simple as there are several areas where deviations from the plan occur. For instance, some deviations are voluntary (e.g. a driver decides to change the delivery sequence) and others are involuntary (e.g. there is a road closure not captured in the digital map data). In many cases, deviation from the plan starts well before a driver even gets on the road. Therefore, to get better control of fleet performance, retailers need to track “plan” versus “actual” performance. Chris Jones, EVP, Descartes explains.
Defining “Plan” Vs. “Actual” Performance
There are three key points to understand when it comes to plan versus actual performance, and how retail fleet operators can use that information to maximise fleet performance and customer experience.
1. Start with an Optimised Route Plan
Many fleet operators make use of route planning solutions to support with retail and ecommerce deliveries. Today’s advanced route optimisation solutions are very adept at considering all the business constraints and evaluating the tradeoffs between having specific orders on particular routes, and the sequence that they are delivered in. They look holistically to find the best combination of routes and sequences that will meet customer delivery requirements for the lowest delivered costs.
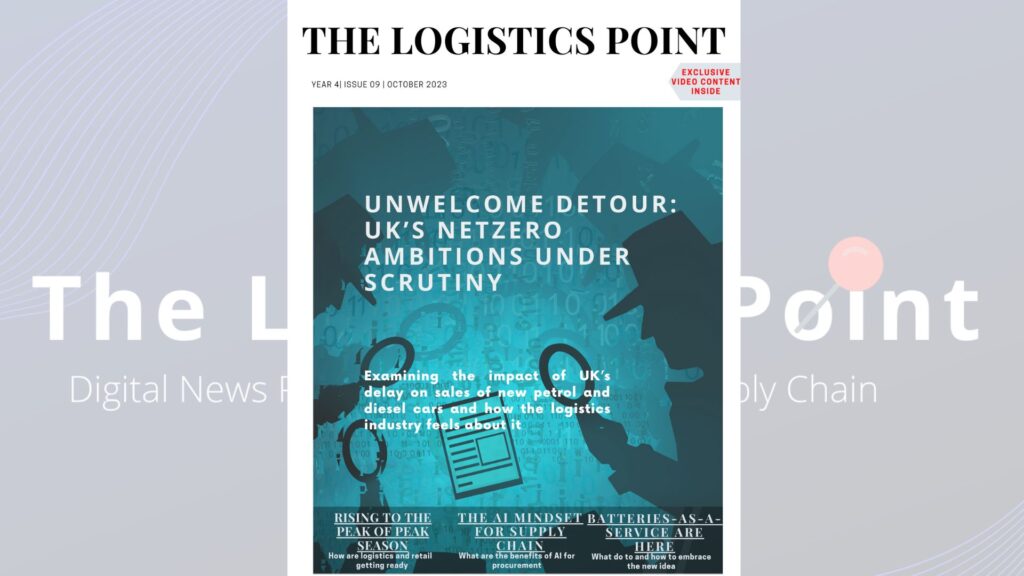
While not perfect, if the route planning solution is configured correctly, it will consistently outperform the human mind to find the most cost-efficient route plan. For this discussion, let’s consider that the plan initially generated by the system is the starting measuring point and has the best potential results.
2. Evaluate the Impact of Changes Made by Planners
Once a plan is initially created it is typically reviewed by a planner to ensure there are not any inconsistencies that could impact delivery performance, and account for any conditions that were not considered in the system configuration, or not possible to model. This step is the first place where deviation from the initial optimised plan can occur.
For legitimate and arbitrary reasons, planners make changes to optimised routes. For instance, the planner knows that the solution doesn’t fully capture a constraint and the number of deliveries that a specific truck can execute.
Equally, a planner may have preconceived notions about what a route should “look like” and make changes to have it appear in a certain way on the digital map. In either case, the optimised plan has been adjusted and the results fall into two categories: more optimised and better performance or less optimised and lower performance. These changes need to be captured and compared to the initially optimised plan.
3. Track Execution and Capture Deviations that Impact It
Once the planner is finished making their adjustments, the plan is published to the driver. Let’s assume that all the deliveries are on the truck and the driver starts executing the route, which is tracked by GPS. Here again, the driver can deviate from the route plan for legitimate and arbitrary reasons.
The driver knows that a certain customer will take orders earlier than indicated in the route plan and changes the delivery sequence to be more efficient, or the driver likes to stop at a specific location because the facilities are better or the food and beverage options more appealing. Then there are events that are out of the driver’s control, which can change the route plan. For example, a customer cancels a delivery or an accident closes a road. All the driver changes and external events need to be captured to get a complete picture of the deviations during route execution.
The Complete Plan Versus Actual Picture
Capturing these points gives fleet managers a comprehensive view of plan through actual execution, and better control of performance outcomes. Managers will know the plan’s starting point in terms of cost and customer service, how the planner’s changes impacted cost and service, and the same for the driver’s changes and external events – all important factors to consider with rising costs for home delivery and fleet operations.
Placed side-by-side, the manager can see (1) if the plan was not as optimal or feasible as possible, (2) the degree to which planners are changing the original plan and why and the degree drivers are deviating and why. With this information, managers can take corrective action to (1) improve the quality of the initial optimised plan through configuration changes, (2) identify which planners are over-editing the plan and negatively impacting costs and customer service, and (3) better manage driver adherence to plan and understand the degree that external events are impacting delivery performance.
Technology Can Refine and Optimise the Review Process
Technology is advancing efficiency across the retail sector continually. It can make the home delivery plan versus actual review process easier, eliminate some of the causes of deviation to plans that have a negative impact on performance; but it can also take into account deviation that had a positive impact. This is how technology supports.
- Data analytics integrated with the planning and execution solution can accelerate the plan versus actual performance analysis. One of the biggest challenges is collecting, organising, and correlating the tremendous amount of data that route planning and GPS-based execution solutions generate.
The advent of powerful, but intuitive and low-cost analytics platforms such as Microsoft PowerBI™ that has standardised integration to route planning and execution solutions streamlines the data management process and gives deep insights into plan versus actual performance.
- How machine learning helps capture plan versus actual performance. Tracking fleet plan versus actual delivery performance is an excellent application for machine learning because of all the data that is created in the route planning through execution process.
Machine learning can more accurately identify actual stop location, drive, service and stop times, and other patterns such as changes in stop sequence. These recommendations can be applied to the optimised planning solution to create more accurate and productive route plans.
Machine learning can also identify which planners and drivers are outliers to capture best practices or coach poorer performers. - Robotic process automation can eliminate some of the causes of plan versus actual deviation. Unfortunately, planner performance can vary widely resulting in significant deviations to the initial optimised plan and poorer plan performance.
By capturing and automating the planning practices of the best planners using robotic process automation, fleet operators can eliminate many of the post-optimisation tweaking that occurs during the planning review phase. Consequently, there will be fewer changes, more predictable planning outcomes across the organisation, shorter planning reviews, and greater planner productivity.
Conclusion
Plan versus actual delivery performance analysis is an important process for pinpointing and improving the practices and actions that planners and drivers take that negatively impact home delivery performance for retailers.
Using this three-point approach described allows managers within retail and ecommerce organisations to capture the changes that impact home delivery performance. When combined with technological advances such as data analytics, machine learning, and robotic process automation, fleet operations can implement powerful plan versus actual performance processes that drive delivery fleet performance and improvements to the bottom line.