In today’s rapidly evolving business landscape, logistics demand forecasting stands as a pivotal element in staying ahead of the curve. The ability to foresee future trends, driven by comprehensive data analysis and a deep understanding of market conditions, becomes a game-changer.

The integration of AI-driven forecasting models promises to usher in a new era of precision and foresight. By leveraging machine learning algorithms and advanced analytics, AI empowers organisations to decode complex patterns, mitigate uncertainties, and potentially gain a more nuanced and accurate view of demand dynamics.
‘’Logistics demand forecasting is a crucial step for us, predicting future trends based on data and market conditions can help us understand and predict demand more accurately. The use of AI forecasting will revolutionise this part of the logistics process in the future, helping organisations potentially get a more accurate view of demand,’ explains Lucie Hyde, Managing Director at LNH Transport.
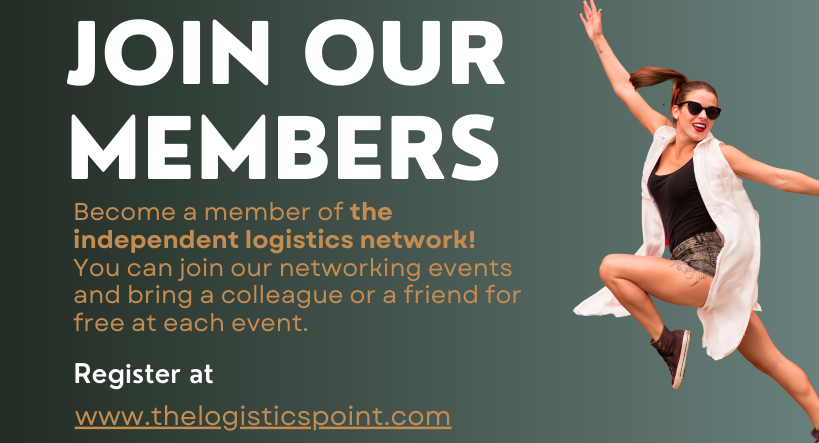
Impacting all areas
AI has also had a profound impact on warehouse operations. Alongside technologies like robots and automated systems, processes such as picking and packing, inventory tracking as well as worker safety have been streamlined, resulting in increased productivity, and reduced human error.
Predictive maintenance has been another transformative aspect. AI’s ability to analyse data from sensors and various other sources means that it can now anticipate maintenance requirements, ensuring minimal downtime and optimal equipment reliability. Lastly, in terms of risk management, AI’s capability to sift through data from myriad sources allows it to pinpoint potential risks and vulnerabilities in the supply chain.
The good and bad
AI boasts benefits like heightened efficiency, accuracy, cost reduction, and superior decision-making. It automates tasks, analyses data, and provides predictions, fostering quicker and more precise decisions, decreased errors, and heightened productivity.
‘However, drawbacks include potential job displacement, ethical dilemmas, and substantial investment needs. Automation may replace human jobs, raising ethical concerns such as bias and privacy issues. Implementing AI demands significant financial investments in hardware and software, acting as a potential hurdle for some organizations,’ says Iterate.
‘Understanding that there is a balance to adapting AI requires educating managers about these technologies from reliable sources and not just the internet to make informed decisions. This requires a mindset shift and a willingness to invest in training and development for companies in the supply chain which they haven’t done traditionally,’ agrees Nilay Parikh at Arvist.
The ability for AI to minimise human error in the logistics sector is groundbreaking, but there is a risk that people will become too reliant on this technology. Although errors can be mitigated, AI is still a developing technology that should not be heavily relied upon, but instead used as a tool to make processes smoother and safer.
“The use of AI in logistics aims to reshape numerous sectors including the dairy distribution industry, by significantly decreasing the risk of error in supply chain documents,’ shares Paul Lawrence, Managing Director of AddSecure UK, North America and Australia.
Return on investment
Putting a price tag on AI investments has to be done effectively and continuously. ‘You don’t want to buy a software solution that you then need to hire consulting services to help correctly configure or end up investing in supply chain engineers and data scientists to help orchestrate it, product teams to manage business requirements, etc,’ argues Alla Anashenkova, Head of Product and Sales Engineering at ThroughPut Inc.
A study conducted by McKinsey found that companies that fully embrace AI in their supply chain operations could see a 10-20% increase in annual revenue. We have seen our customers at Arvist save over $300,000 annually by implementing a simple quality control application using AI. There are various variables around the potential savings, and it varies from use case to use case, so it is important to identify potential value that an AI solution can bring before implementing.
While it may be difficult to quantify the exact ROI of AI projects in this field, the potential for positive impact is clear. The ability to increase efficiency, reduce labor expenses, and improve customer satisfaction are all tangible benefits that can need to be taken into account while calculating the ROI of AI,’ adds Nilay Parikh.
The cost of an AI project can be broken down into several categories, including hardware, software, data, and personnel costs. Hardware costs include the cost of servers, storage devices, and other hardware required to run AI models. Software costs include the cost of developing or purchasing AI software, as well as any necessary licences Data costs include the cost of collecting, storing, and processing large amounts of data required for AI models. Personnel costs include the cost of hiring and training AI experts, data scientists, and other personnel required for the project.